Consistency in AI Skin Analysis: Why Reliable Results Build Trust
Consistency in AI skin analysis ensures reliable and repeatable results, building trust in technology. Factors like lighting, camera quality, and user behavior affect outcomes. Solutions include robust processing, user guidance, and continuous improvement for dependable performance.
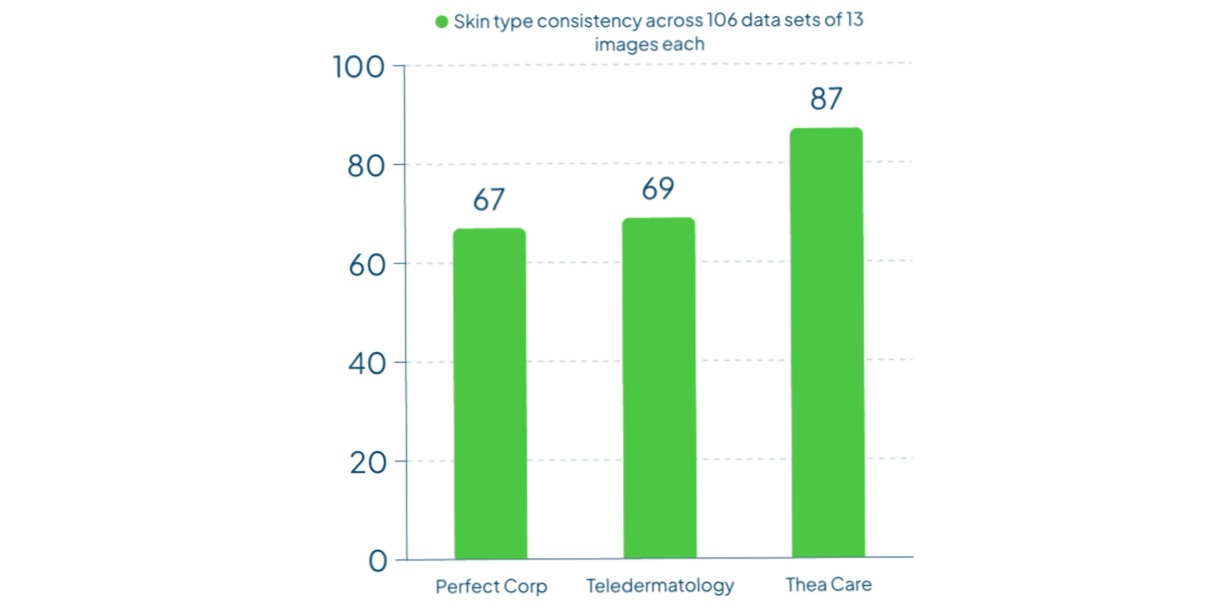
1. Introduction
The world of skincare is experiencing a genuine boom in innovative technologies. Whether in e-commerce or brick-and-mortar stores, many companies are now leveraging AI-based skin analyses to offer personalized recommendations to their customers. However, despite the excitement around artificial intelligence, there is one crucial factor that determines the success of such solutions: consistency.
In this context, “consistency” means that the skin analysis does not produce completely different results under minimally changed conditions. Imagine someone performs the analysis twice in a row and gets “dry skin” as a result once and “oily skin” the next. This discrepancy not only confuses customers but also severely undermines trust in the AI technology.
In this blog post, you’ll learn why consistency in AI skin analysis is particularly important, which factors influence stability, and how companies can ensure that their skin analysis delivers reliable results over the long term.
2. What Does Consistency Mean in AI Skin Analysis?
Consistency stands for repeatable and stable results under similar conditions. For example, if the same person performs the skin analysis shortly after the first attempt without significantly changing factors (lighting, distance, camera angle, etc.), the results should largely match.
- No radical deviations: Minor differences are normal, such as in the assessment of moisture levels. Major shifts—like from “very dry” to “oily”—are a clear warning sign.
- Same or similar product recommendations: If a user is recommended a hydrating serum once and a clarifying anti-acne product the next time, without any objective change in their skin condition, the solution seems unreliable.
Especially in e-commerce, a lack of consistency leads to a loss of trust—a bad outcome for everyone involved. After all, one of the main arguments for AI-based skin analysis is its promise of being customer-specific and reliable.
3. Factors Influencing Stability of Results
3.1 Lighting Conditions
Lighting is a significant disrupting factor. Different light intensities or directions can either highlight or conceal skin imperfections.
- Intensity: In a dark room, wrinkles or shadows appear more pronounced. On the other hand, overly bright light can make the skin look smoother.
- Direction: Backlighting can distort facial contours, while side lighting emphasizes shadows.
- Outdoor vs. Indoor: Natural daylight differs significantly from artificial lighting. Even the time of day can have an impact (softer light in the morning, harsher light at noon).
3.2 Device and Camera Quality
A simple laptop webcam delivers different color tones and sharpness levels than a modern smartphone camera.
- Resolution: Low resolution can cause critical details, like fine wrinkles or subtle redness, to be lost.
- Automatic Image Optimization: Some devices activate beauty modes or HDR features, manipulating the image.
- Hardware-Driven Color Deviations: Different camera manufacturers calibrate their sensors differently, leading to varying skin tones.
3.3 User Behavior
How the person stands, sits, or looks into the camera also matters.
- Face Angle: A slight head tilt can change the visibility of certain facial areas, such as under-eye circles or cheekbones.
- Distance: Standing too close or too far from the camera results in different image sections being captured by the algorithm.
- Facial Expressions: Smiling in the first attempt and frowning in the second can highlight different wrinkles.
All these influences make it more or less challenging for AI to extract true skin parameters and consistently generate reliable results.
4. Consequences of Inconsistency
When results vary significantly despite minimal changes, it has consequences for both end users and B2B companies:
- Loss of Consumer Trust: Those who receive different results no longer believe in the “intelligence” of the technology. Next time, they may prefer traditional methods or regard the entire system as mere gimmickry.
- Doubts About the Technology: For brands investing in AI solutions, lack of reliability poses a problem. Ultimately, this technology becomes part of the brand’s promise.
- Negative Customer Reviews: In the age of social media, bad experiences spread quickly. Inconsistent skin analysis risks poor reviews and reduced recommendations.
5. Measures to Ensure Greater Stability
5.1 Technical Optimizations
- Robust Image Processing: AI developers should ensure algorithms are calibrated for various lighting conditions. A form of “light balancing” can correct extreme exposure differences and color distortions.
- Quality Checks: A pre-check could warn users if the image is blurry or if the face is only partially within the frame. Some systems even detect whether a person is too close or too far from the camera.
- Device-Agnostic Standards: A broad testing phase on common smartphones, laptops, and tablets helps mitigate differences between cameras. Regular updates keep the system up-to-date.
5.2 User Guidance
- Lighting Tips: Short suggestions before the analysis (“Please stand in front of a window with sufficient daylight.”).
- Distance Recommendations: A scale or frame within the camera feed can indicate whether the face is at the right distance.
- Simple Step-by-Step Guides: A pop-up guiding users through the process helps avoid incorrect usage. For instance, unnecessary movements or changes in facial expression during the critical moment can be minimized.
5.3 Internal Processes & Quality Assurance
- Regular Test Runs: An internal team or test users can assess the AI under different scenarios (indoor, outdoor, different camera types) and provide feedback.
- Continuous Model Improvement: With each iteration, the AI model learns more. It is crucial to have a data management process in place that incorporates feedback loops.
- Monitoring & Reporting: The system should be continuously monitored. A sudden surge in “erratic results” may indicate a software bug or a poorly trained algorithm.
6. Conclusion
Consistency is the cornerstone when it comes to implementing AI skin analysis in the B2B or D2C space. Only when users achieve similar results across multiple attempts will the technology earn their trust. A combination of technical robustness and clear user guidance is essential. For e-commerce brands, this means that a one-time integration of skin analysis is not enough. Continuous observation of results, ongoing testing, and constant fine-tuning are required. Those who follow these steps will benefit from satisfied customers who trust the AI—and thus strengthen their connection to the brand.